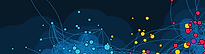
Graph Algorithms for Imaging and Networks GAIN 2015
Important Dates
Organizers
Blake Hunter
Claremont McKenna College
Mathematical Sciences
Yves van Gennip
The University of Nottingham
School of Mathematical Sciences
Paper submission deadline:
Jul 20, 2015
Paper acceptance notification:
Sep 1, 2015
Workshop date:
Nov 14, 2015
Graph Algorithms for Imaging and Networks
(GAIN 2015)
Recent years have seen many advances in graph techniques for image segmentation, data clustering, and community detection in social networks. As the graph size and dimension of the data grow, they create new feasibility and computational challenges for partitioning and analyzing data. A wide array of mathematical techniques have been developed to solve these issues including spectral methods, random sampling, dynamics on networks, and variational methods.
Not only is the curse of dimensionality an obstacle to clustering, but high dimensional geometry suggests that samplings of high dimensional data tend to live mostly on the boundary of clusters, complicating graph partitioning even further.
Image segmentation, data clustering, and community detection all can be approached via graph partitioning techniques, yet application specific demands often require unique graph constructions and algorithms and high level interdisciplinary expert collaboration. This workshop brings together such experts, with backgrounds in graph theory, network analysis, computer science, image processing, and analysis of partial differential equations.
Topics of Interest
1 New algorithms for fast, large-scale and online graph partitioning
2 Improved theoretical and mathematical understanding of existing or new graph partitioning algorithms and their connections
3 Strategies to deal with high dimensional data
4 Applications of graph partitioning to image segmentation
5 Applications of graph partitioning to data clustering and classification
6 Applications of graph partitioning to community detection in social networks
7 Novel applications of graph partitioning